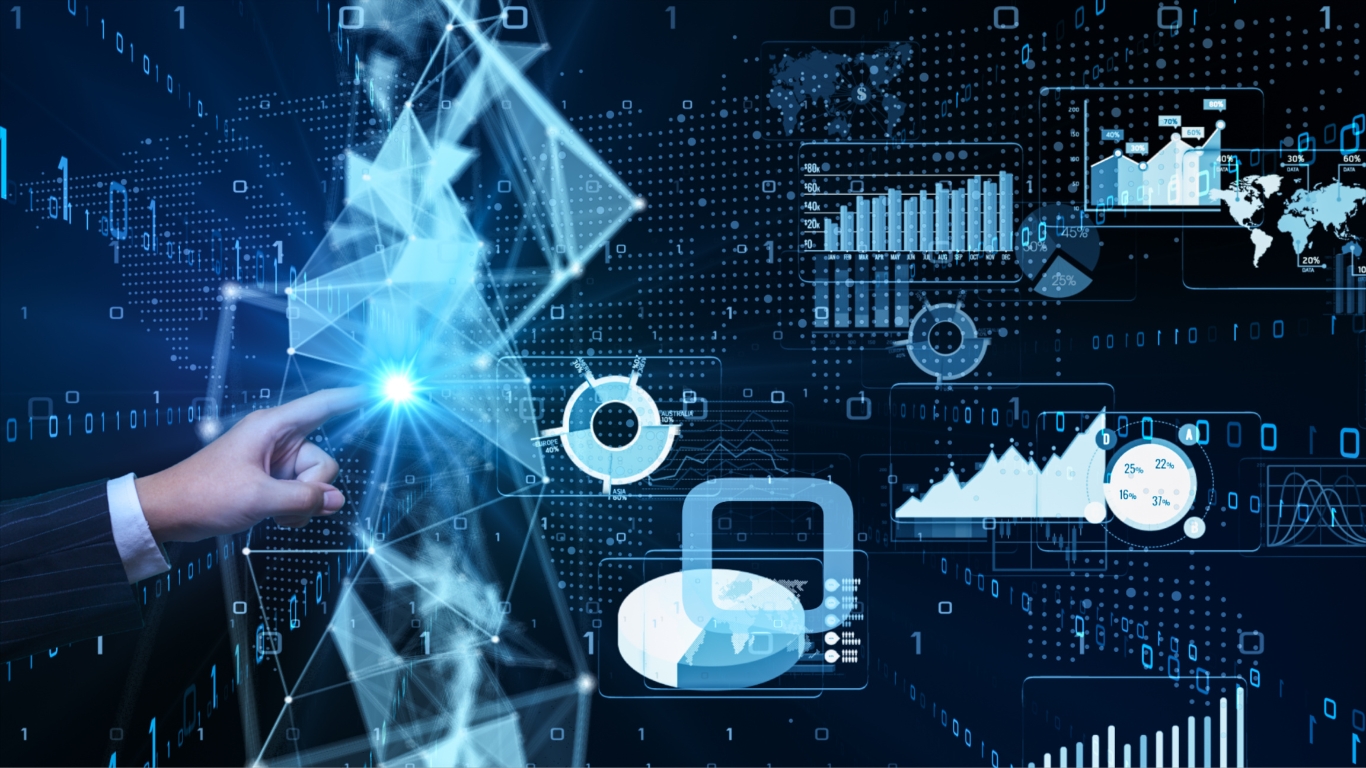
Written by Professor Ignacio Cofone
The Information Economy and the AI Turn
At Facebook’s initial public offering in 2012, Mark Zuckerberg shared a motto that would then turn into a mantra: “move fast and break things.” While Facebook has since abandoned it, its ethos remains central both to how AI innovation is often justified – seeing disruption as inherent to progress – and to critics who decry the social costs of unchecked innovation. But the uncomfortable truth is that we designed our legal systems to reward this kind of behaviour. In the information economy, as long as companies and government entities comply with formal commitments and check the right regulatory boxes, the social consequences of their practices usually go unaddressed. If we want a future in which AI developers and platforms don’t just “move fast and break things,” we should align expectations and incentives. That means making it costly to break things that matter.
The information economy refers to a system in which companies generate profit not only from direct money transactions, but also from our data. AI supercharged this system by allowing to transform enormous amount of raw personal data into useful and profitable inferences, classifications, and behavioural forecasts.
These uses lead to downstream harms that, unlike most harms from collected data, are impossible for individuals to foresee. Take the growing use of generative AI to create deepfakes. In 2023, an Australian man created and distributed non-consensual deepfake pornography featuring real women – including students and teachers – despite court orders to desist. In 2024, a teenage girl in New Jersey sued a classmate who used generative AI to make and distribute explicit images of her. Just a few years ago, courts may have dismissed such cases as unfortunate consequences of freely shared data. But the harms these individuals experienced weren’t incidental – they were structural. They were the result of these individuals being pulled into the information economy. While the creators of abusive content bear responsibility, so do the services that enabled and profited from the production and amplification of such content.
AI harms in the new information economy, which is an inference economy, rarely stop at one individual. Many predictive policing systems, for example, disproportionately misclassify individuals from racialised and marginalised communities, amplifying existing inequities beyond any individual false arrest. AI recruiting tools are sometimes found to systematically disadvantage women due to biased training data. AI-driven personalisation in data-driven political campaigns implicate collective democratic processes. Every single person is exposed to the harms of the new information economy, regardless of their individual choices, because interactions that involve personal data are an indispensable part of our lives.
Consumer Protection in the Inference Economy
Current data protection and privacy frameworks are ill-fitted for an AI-powered information economy. They largely follow a consumer protection model based on outdated assumptions: one-on-one interactions between individual users and a company where the users can evaluate consequences to choose which practices they consent to. That model no longer fits for three reasons.
The first reason is inference capability: AI processing enables knowing far more about people than what they disclose. Uber estimates optimal pricing and ride demand by analysing location data, weather patterns, and even phone battery levels. Spotify builds personality profiles from listening behaviour. Life insurers and employers use data from wearables to assess risk or productivity. Large language models (LLMs) trained on online forums learn to predict mental health traits or political views from writing patterns. Even public agencies, such as the U.S. Department of Homeland Security, infer security threats by analysing social media activity. None of these inferences are covered by traditional protections such as individual consent to terms of service. Users may agree to sharing their location or activity data, but they can’t really consent to the indeterminate conclusions drawn from them because they can’t predict these conclusions when clicking “I agree.”
The second reason is that personal data processed by AI is relational. AI’s inference capability doesn’t operate on separate silos of individual data and individual inferences but on group correlations and patterns derived from the behaviour and information of others. Large-scale recommender systems on platforms like TikTok or Instagram, for example, use demographic clustering to infer what users might watch, purchase, or believe. Even those who never used a platform aren’t anonymous to it due to how informative the behaviour of similar users is. This means that every time any one person shares data, they also reveal information about others that share connections or traits with them.
The third reason is a change in power structures. AI systems make decisions that were once reserved for individual human discretion. Consider tools like credit-scoring algorithms, which assess the eligibility of loan applicants with minimal human oversight, or resume screening tools that use natural language processing to rank candidates, only some of which will be seen by a recruiter. Similarly, consider AI risk assessment algorithms that estimate how risky a criminal defendant might be to offend to influence bail and parole decisions. These systems do more than replicate human decision-making – they shift the locus of power. What once resided in interpersonal judgment now lies with model developers and data engineers whose decisions shape lives at scale. This shift makes individual choices less relevant and centralises control in ways that are enabled by inference capabilities. Not only the outputs of commercial AI products that one can use matter for people’s lives, but the structure of inference and decision-making that AI imposes does too.
Toward a Model of Meaningful Accountability
Data protection laws remain tethered to outdated notions of risk. Companies are generally held accountable only when they violate something they promised in a privacy policy or when they fail to comply with pre-defined rules – such as failing to conduct a Data Protection Impact Assessment or appoint a Data Protection Officer. But these mechanisms only capture a subset of the risks AI systems pose. Most AI harms don’t depend on a flatly unfair practice or technology that can easily be prohibited; they depend on what’s inferred and how the data will be used down the line. So the deeper harms – such as those stemming from harmful inferences and systemic bias – often fall outside these frameworks. As long as companies and government entities are only required to follow these narrow rules, which they helped shape, they will remain insulated from meaningful accountability.
Adequate AI accountability requires a system that captures the unpredictable consequences that result from inference capabilities, relational data, and changed power structures. Such an accountability system is new for data but it’s not new for the law: similar approaches exist in many other areas. Consider driving. Traffic regulations impose obligations (speed limits, working headlights), but drivers can still be held liable for harm even if they complied with those rules based on the flexible principle of negligence. Because not all risks can be regulated in advance, the accountability framework relies on retrospective judgment to fill in the gaps.
AI governance requires accountability frameworks like these, which recognise the difference between rule-following and harm-avoidance. Developing them involves setting up principles of accountability that respond to the uses of data, not just their collection, and that consider the downstream consequences of model deployment as well as model development. This means conceiving of AI responsibility less as a series of checklists and more as a continuous and adaptive process that grapples with residual uncertainty and power imbalances.
Encouragingly, some regulatory frameworks are slowly beginning to take on this challenge. For example, the NIST AI Risk Management Framework includes context-aware evaluation of potential harms. Yet as a voluntary standard, it relies on institutional will and market pressures for adoption – conditions that may not materialise in sectors where harms are diffuse. The EU AI Act, more cautiously, introduces a tiered risk classification system that focuses regulatory attention on “high-risk” applications. Although the Act is too narrow in defining high levels of risk, it seems to leave the door open to considering responsibility for harms that emerge despite rule-compliance.
Overcoming the Privacy Fallacy
Central to this accountability reform for AI and data is overcoming what one might call the privacy fallacy: the contradiction in simultaneously holding the belief that privacy is an important social value and the belief that people’s privacy matters only when its loss leads to tangible harm like identity theft or financial loss. This view leads to confusions such as that people who have “nothing to hide” have nothing to fear (because privacy is reduced to the negative material consequences that it prevents).
But people’s privacy isn’t merely instrumental. It underpins dignity, as the freedom from being instrumentalised through one’s data, and autonomy, as the ability to function without behavioural manipulation. AI systems that infer people’s emotions, preferences, or vulnerabilities – whether for insurance pricing, political persuasion, or consumer targeting – solely to manipulate them undermine that foundation. So do AI systems that process personal data to turn people’s racial or gender identity against them in biased decision-making systems. They do so even if they don’t also harm their bodies and their wallets.
Protecting people in an AI society requires us to value not only tangible outcomes, but also the social values that affect people’s lives. In the new information economy, where AI systems increasingly structure access to social and economic opportunities based on people’s data, meaningful accountability for data uses is essential. That means recognising that our current rules were built for a different economy, and that reform begins with an assessment of how data, business models, harms, and power now interact.
This blog post has been prepared as a summary presentation of ideas developed in The Privacy Fallacy: Harm and Power in the Information Economy (Cambridge University Press, 2023).
Suggested citation: Cofone I., ‘Why AI Harm Escapes Accountability in the New Information Economy’, AI Ethics at Oxford Blog (15 May 2025)